Connected technology is the backbone of data transfer – data that can be used to predict failures and optimize workflows to enhance industrial operations.
The automation landscape has evolved from isolated programmable systems to an interconnected network of intelligent devices. The integration of the Internet of Things (IoT) has changed much of the technology landscape, enabling a seamless flow of data across various systems. Thisindustrial transformationhas facilitated a more nuanced control and coordination, allowing for a dynamic response to operational demands.
IoT has profoundly impacted robotics maintenance. It has transformed maintenance from a siloed task into an integrated aspect of operations. Real-time data from sensors on robotic units can be used to provide immediate diagnostics, preempt failures, and schedule maintenance tasks more effectively, reducing downtime and maintenance costs.
This article will explore the ways in which the connected technology of the IoT movement is transforming robotic maintenance.
Shifting Maintenance Paradigms
The transition from reactive to proactive maintenance, catalyzed by IoT, involves a fundamental shift in approach. IoT now enables maintenance teams to predict equipment failure before it occurs, using advanced analytics on sensor data to identify patterns indicative of mechanical abnormalities.
Traditional maintenance regimens, often based on calendar dates or usage hours, lack the precision of IoT-enhanced approaches. IoT enables a dynamic maintenance schedule, driven by real-time equipment performance data. This shift not only reduces maintenance costs but also improves equipment availability and reliability.

Technicians working on automated equipment
Figure 1.Predictive maintenance strategies can solve failures even before they happen. Image used courtesy ofAdobe Stock
Harnessing Real-Time Data for Predictive Maintenance
Incorporating data analytics into robotic maintenance strategies optimizes fault detection and operational efficiency. Advanced algorithms analyze data patterns to predict failures, allowing maintenance in advance of failures. This reduces unplanned downtime and extends the lifecycle of robotic systems.
Effective implementation of predictive maintenance in robotics requires a strategic blend of data analysis and operational planning. This includes deploying machine learning algorithms for anomaly detection and establishing protocols for maintenance actions based on predictive insights. It’s a balance of technological integration and operational foresight.
Real-world applications, where IoT-driven predictive maintenance has been implemented, demonstrate significant operational improvements. IoT's role in improving maintenance is exemplified by manycase studies across different industries. These examples demonstrate a significant shift in maintenance strategies, with one study revealing a reduction in unplanned downtime by up to 50% through the adoption of IoT-driven predictive maintenance.
This showcases how real-time data collection and analysis enable businesses to anticipate and address maintenance needs before they escalate into larger issues. The integration of IoT into maintenance practices not only minimizes downtime but also extends the lifespan of equipment, leading to substantial efficiency gains and cost savings for organizations.
Operational Optimization with IoT
The benefits of optimizing operations through IoT extend beyond predictive maintenance into real-time operational enhancements. IoT also enables:
Adaptive scheduling:This method utilizes IoT data to dynamically adjust work schedules, aligning resources with demand fluctuations.
Dynamic resource allocation:IoT informs the allocation of resources, optimizing their use based on real-time operational needs.
Process adjustment in real time:IoT enables immediate responses to changing conditions, enhancing workflow efficiency.
Bottleneck reduction:By analyzing data patterns, IoT identifies and addresses workflow bottlenecks, streamlining operations.
Strategic operational optimization with IoT requires integrating data insights into every aspect of production planning and execution. This includes adopting a data-centric approach to workflow management, resource allocation, and quality control, thereby ensuring peak operational efficiency.
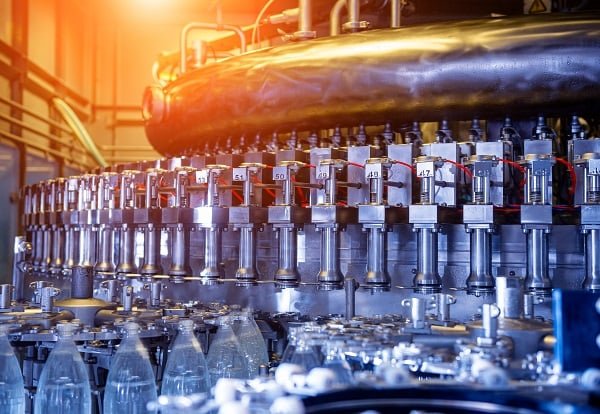
Production bottle-filling line
Figure 2.Throughput can be increased by analyzing the sources of production bottlenecks. Image used courtesy ofAdobe Stock
A critical evaluation of IoT's impact on production involves deep analysis of throughput, quality control metrics, and machine efficiency. This data-centric evaluation helps in pinpointing areas for improvement, aligning maintenance strategies with production goals, and optimizing operational workflows for better outcomes.
Life Extension and Safety Enhancement
Prolonging equipment life with IoT involves using data to fine-tune operational parameters and preempt wear and tear. Strategies include adjusting operational loads based on real-time performance data and scheduling maintenance activities to address early signs of deterioration.
IoT enables advanced safety protocols in robotic environments by facilitating real-time hazard monitoring and automated emergency response mechanisms. This proactive safety approach minimizes risk and ensures compliance with evolving safety standards in industrial settings.
Industrial applications of IoT technology cancreate safer work environments. Examples include the use of computer vision to monitor workplaces in real-time, sensors to alert for equipment maintenance needs, RFID tags for personnel safety, wearable technology for monitoring workers' health signs, and augmented reality (AR) applications for complex equipment maintenance.
All of these technologies contribute to safer workplaces and longer equipment lifecycles.
Building a Resilient and Adaptive Manufacturing Ecosystem
When building a resilient manufacturing ecosystem, IoT serves as the backbone for strategic intelligence. It enables a seamless flow of data across the manufacturing chain, facilitating informed decision-making and rapid adaptation to changing conditions.
Adapting to rapid market changes requires a robust data framework that supports agile decision-making. This involves:
Real-time monitoring of production metrics
Quick integration of market feedback into production planning
Flexible manufacturing processes capable of adapting to changing demands
These framework components help industrial organizations build resilience by fostering adaptability.
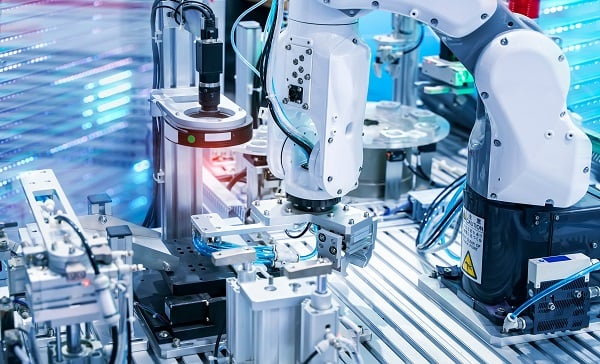
Robot assembling a device
Figure 3.IoT applications require a balance between physical data and operational goals. Image used courtesy ofAdobe Stock
IoT in Action: Practical Insights and Applications
Transitioning from theoretical IoT models to practical applications requires a deep understanding of both technological capabilities and operational contexts. Real-world applications, such as automated quality control and real-time supply chain optimization, demonstrate the practicality and benefits of IoT in industrial settings.
For seasoned professionals, mastering IoT involves not just understanding its technical aspects but also its strategic implementation. This includes leveraging data for advanced decision-making, integrating IoT with other emerging technologies, and continually evolving IoT strategies to anticipate emerging trends and integrate cutting-edge advancements, ensuring a competitive edge in digital and technological innovation.
Addressing challenges in IoT implementation, such as data security and integration complexities, requires a sophisticated approach that is multi-faceted, encompassing adept data management, regulatory compliance, user-centric design, and a commitment to continuous improvement and innovation.
Looking Ahead: The Future of IoT in Industrial Robotics
Staying ahead in the field of IoT in industrial robotics involves anticipating and adapting to emerging trends such as increased automation, the integration of AI, and the development of more sophisticated IoT solutions. Being proactive in this adaptation is key to leveraging the full potential of IoT in the industry.
Preparing for technological shifts in IoT entails continuous investment in R&D, upskilling the workforce, and maintaining a flexible operational framework. This preparedness is crucial for swiftly integrating new technologies and maintaining a competitive edge.
Fostering a culture of innovation and continuous learning is vital for keeping pace with advancements in IoT and robotics. This includes exploring new IoT applications, continually refining processes based on data-driven insights, and embracing technological changes as opportunities for growth and improvement.
As we look towards the future, the integration of IoT in industrial robotics and maintenance is not just a trend, but a paradigm shift shaping the fabric of manufacturing and production. The convergence of advanced analytics, real-time data monitoring, and intelligent decision-making heralds a new era of efficiency, safety, and resilience.
RELATED CONTENT
Potential Applications and Challenges for Blockchain in Industrial Automation
Introduction to Industrial Barcode Reading
Tutorial on Industrial Signal Conditioning
Addressing the Speed and Power Requirements of Automation Controls with Rugged Solutions
Siemens Launches the Open Industrial Edge Ecosystem to Promote Data-driven Industrial Automation